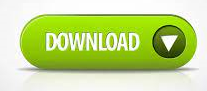
In sum, our results demonstrate the deep unity of the processes and patterns of cultural and organic evolution.

We suggest that these forces are probably cultural selection and that the evolution of many artefact traits can be explained by a shifting-optimum model of cultural selection that, in turn, rests on known psychological biases in aesthetic appreciation. Using time-series methods, we show that much of modern culture is shaped by either stabilizing or directional forces or both and that these forces partly regulate the rates at which different traits evolve. In contrast to the general belief that modern culture evolves very quickly, we show that rates of modern cultural evolution are comparable to those of many animal populations. Here we investigate the evolutionary dynamics of several kinds of modern cultural artefacts-pop music, novels, the clinical literature and cars-as well as a collection of organic populations. While this paper is limited to Spotify data, it underscores the potential contribution of machine learning in exploratory approaches to research on cultural differences. A follow-up study using Top-50 charts found similarly significant differences between cultures for these three features.Ĭonclusion: We demonstrate that machine learning can reveal both the magnitude of differences in music preference across Taiwanese, Japanese, and American markets, and where these preferences are different. Model interpretation revealed music features of greatest importance: Overall, popular music in Taiwan was characterised by high acousticness, American music was characterised by high speechiness, and Japanese music was characterised by high energy features. Accuracy scores for binary classification models ranged between 0.71 to 0.81. Results: The multiclass models achieved moderate classification accuracy (GBDT = 0.69, MLP = 0.66). To ensure the reliability of our interpretations, we conducted a follow-up study comparing Top-50 playlists from Taiwan, Japan, and the US on identified variables of importance. We use both multiclass classification models (Gradient Boosted Decision Trees (GBDT) and Multilayer Perceptron (MLP)), and binary classification models, and interpret their results using variable importance measures and Partial Dependence Plots.
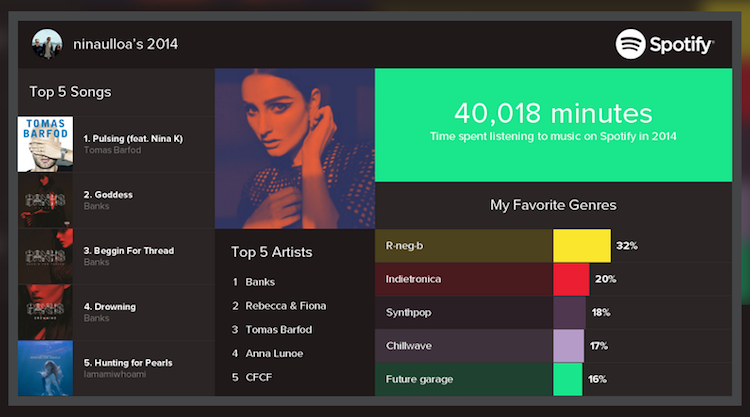
Methods: We present an exploratory analysis of 1.08 million songs centred on Taiwanese, Japanese and American markets. In this paper, we demonstrate that machine learning classification on cultural market membership (Taiwanese, Japanese, American) by music features reveals variations in popular music across these markets. The widespread prevalence of music streaming has allowed for music feature information to be consolidated by service providers like Spotify. Background: Preferences for music can be represented through music features.
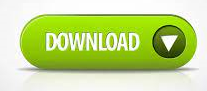